Today, detecting and stopping fraud is possible by identifying the signs and indicators of a potential breach of cyber financial security. Detecting fraud can be a time-consuming and inaccurate procedure if too much emphasis on human work. Due to this, most teams are turning to machine learning to detect fraud.
Machine learning fraud detection can analyse larger datasets for more extended periods without becoming tiring. They can accurately spot warning signals of fraudulent activity before it occurs.
So, anyone keen in know how to use machine learning to detect fraud should keep reading. Because in this blog Sky Potentials UK, the machine learning consultation company, will explore the benefits of using machine learning for fraud protection so that you can make more knowledgeable choices.
What Is Machine Learning In The Sphere Of Detection Of Fraud
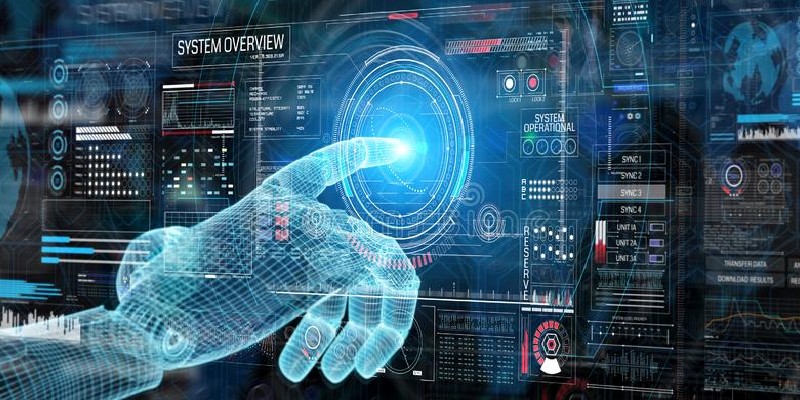
A crucial part of fraud detection using machine learning is using historical data to train machine learning algorithms to differentiate between legitimate fraudulent and perfectly legal actions to detect future happenings.
Supervised learning and unsupervised learning are the two key classes of machine learning.
In supervised learning, a machine learning system begins identifying fraud anonymously. This involves labelling and dividing the information the system requires to study and feed it into the system.
Yet, in unsupervised learning, labelling and partitioning the data is optional. Instead, you give the data to an algorithm, which automatically sorts it into groups. Unsupervised machine learning is valuable for unseen threats. Although, it needs more processing power for the best performance.
Comparing Using Outsourced Fraud Detection Versus In-House Using Machine Learning

There is no generic method to set up a machine learning team in-house or contract it out for your machine learning fraud detection.
Cost: Using an in-house team to develop a unique ML model requires experts, which is costly. While outsourcing requires is like to only pay someone else to perform the risky work for you.
More time to prepare data: There’s a chance you’ll have to do some groundwork to get the data ready for the machine learning model to distinguish between fraudulent and legitimate actions. The procedure is lengthy, and you need more time to complete it. As this is the case, outsourcing would be the most effective option.
Variety of Data Sources: When it comes to internally hiring for machine learning models and fraud detection, you will have insufficient data to establish reliable thresholds and rules, as you only have accessibility to the data that is in your range. In contrast, outsourcing allows you to access more information from other clients and apply that knowledge to your situation for more accurate results.
Ways to explain the operation of a machine learning fraud detector?
Whether you want an in-house team to handle machine learning for fraud detection or contract it out, it is essential to take a series of measures to guarantee that you will not overlook any fraudulent attempt.
Data input for source material: A machine learning algorithm must first receive data to begin forming its beliefs. Feed it with details such as the financial activity origin and the credit card info. Don’t attempt to overload it by including only relevant information.
Produce system rules: Put your machine learning system to execute complex or basic rules for identifying and stopping potentially fraudulent actions involving your financial assets. Here you can select the threshold, the rejection and acceptance rates.
Check the algorithm’s rules and turn them on: After establishing the parameters within which the machine learning algorithm will function, you will need to review those parameters and turn on the algorithm only when you are satisfied that it fulfils your quality standards. You can modify the threshold either by hand or automatically via machine learning.
Educate the algorithm: At first, the algorithm is still figuring out what does and does not qualify as a fraudulent transaction. Thus, when you begin, your team should provide feedback from the machine’s early findings through acceptance, rejection, or revision. With more time, you will have to do this less often.
Sandbox historical testing: You can test in a sandbox environment before successful fraud actions. Here you can test how well your system works by turning the rules on and off.
Different Fraudulent Actions and How Machine Learning Can Stop Them
Let’s have a look at a few examples of fraud detection using machine learning before we go into the fix:
Defrauding The Tax System
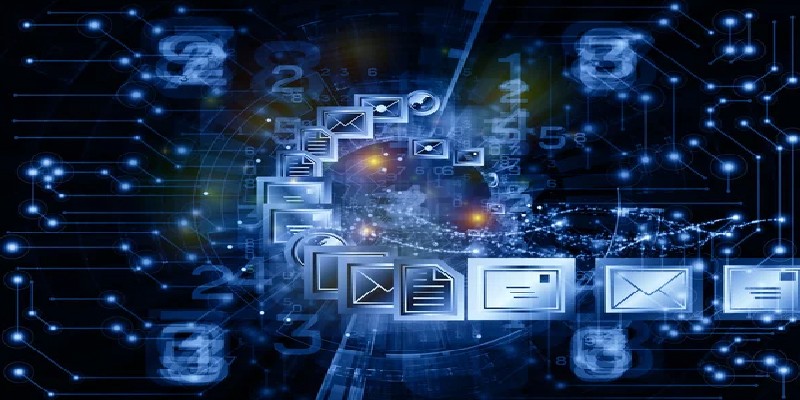
Some people and companies try not to pay their taxes fair share and hide their fortune and show high company expenses. Machine learning algorithms analyse a variety of indicators to determine if a person is being truthful or dishonest.
Insurance-related fraud
Machine learning to detect fraud can access insurance forms and notes that doctors, nurses, and victims fills and decrease claims of fraudulent insurance. It identifies inconsistencies and other filling errors in the forms.
Suspecting someone’s identity
Scheming loan applications and e-commerce scams are examples of identity theft. With the behavioural detection tools, machine learning can recognize suspicious activity.
Credit Card Scams
Machine learning systems can detect credit card fraud, considering factors such as the frequency and value of luxury purchases and recent buying on a card close to the expiration. Another example is the algorithm can check the card if it notices several new purchases or payment methods are made on a card to it in a short time frame. The machine learning system will stop these kinds of transactions from ending the behaviour and prevent it from happening again.
Money Laundering
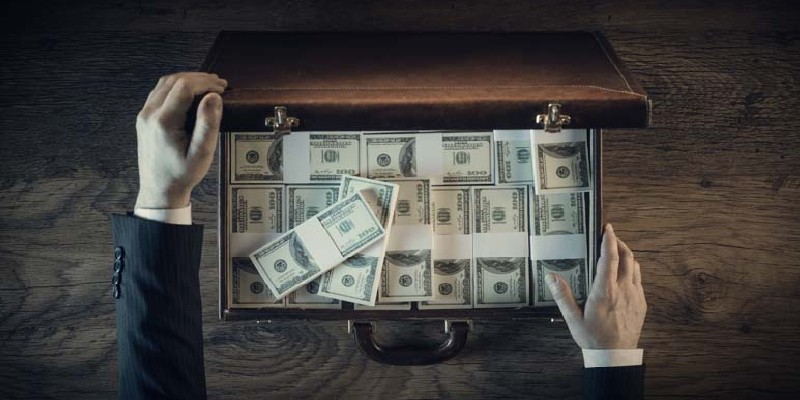
Using machine learning, investigators can distinguish between three distinct sorts of financial transactions: money laundering, the transfer of money that bank flags because of unusual alerts, and those that are everyday lawful transactions. The machine learning system remembers the transaction details, including who sent and received the money and how often, and sounds the alarm if a similar transaction occurs again.
If you are an e-commerce business owner and want to ensure tight security in your transaction process, contact Sky Potentials UK for your business’s strong safety. We are Europe’s top machine learning consultation service and business solutions provider. Make your business more secure with our assistance, as we will always be there for you with our ML expertise.
Manipulation of the Market
When someone intentionally changes market supply and demand for financial advantage, they manipulate the market. Machine learning can avoid market manipulation by monitoring stock-buyer and broker data.
Benefits of Having Machine Learning for Fraud Prevention
Among the many advantages to using machine learning in fraud protection for businesses are:
Algorithms can perform All Time That humans can’t
For instance, when Joe, the fraud detector, clocks off for the day, the fraudsters and their financial crimes don’t stop. Unlike humans, algorithms can function around the clock. In contrast to Joe, machine learning systems do not clock out at the end of the day or take vacations.
The intended lifespan of these systems and algorithms is indefinite. For them, preventing fraudulent actions is their sole concern; they don’t get sick, sleep, or take time off for holidays and personal affairs; thus, they’re more effective than people at their job.
Saving Money With Machine Learning Is Possible
In most cases, machine learning algorithms can help you avoid adding a team to your risk operations department. Some companies’ operations increase at certain times of the year, and it cannot make financial sense to hire extra fraud analysts and fraud detection specialists just for those times.
Business requires more time and energy to help new hires adjust to the company’s culture when brought in at specific times of the year. On the other hand, machine learning systems can handle heavy traffic at any time of day or year. Additionally, the company only needs to scale the system if and when fraud attempts increase.
Larger Datasets Allow For More Precise Forecasting
Before you must predict how something performs, you first view its action. The same holds for the detection of fraudulent activity. The problem with relying exclusively on human labour is that individuals have limited time and energy to process and evaluate data.
Yet, machine learning systems are designed to process more excellent datasets than humans can. Most importantly, machine learning algorithms can effectively anticipate fraud in a shorter amount of time due to their increased capability.
Less Time Spent Manually Reviewing
With machine learning technologies, you can handle manual, time-consuming, and error-prone data evaluation. Machine learning allows an algorithm to process large datasets much faster than a human could.
The solution lies in combining human work and ML. For this reason, a fraud detector won’t have to put in a lot of work performing manual data assessments in coexistence with ML technology. Therefore, it becomes less complex, less time-consuming, and less demanding.
Conclusion
As our technical solutions improve, so will the sophistication of fraudulent transactions and efforts. Despite widespread doubt about the goodness of machine learning and AI, these systems are now the best option for identifying identity theft, credit card fraud, tax evasion, and money laundering. Software and systems can change along with fraudulent attempts only with the human touch of creating rules, thresholds, and reviewing parameters.
Chat with Sky Potentials UK now for solid online security for financial support. As a software development company and business solutions provider, we will always support you in building the most secure and robust business in digitalisation.
Leave a Reply